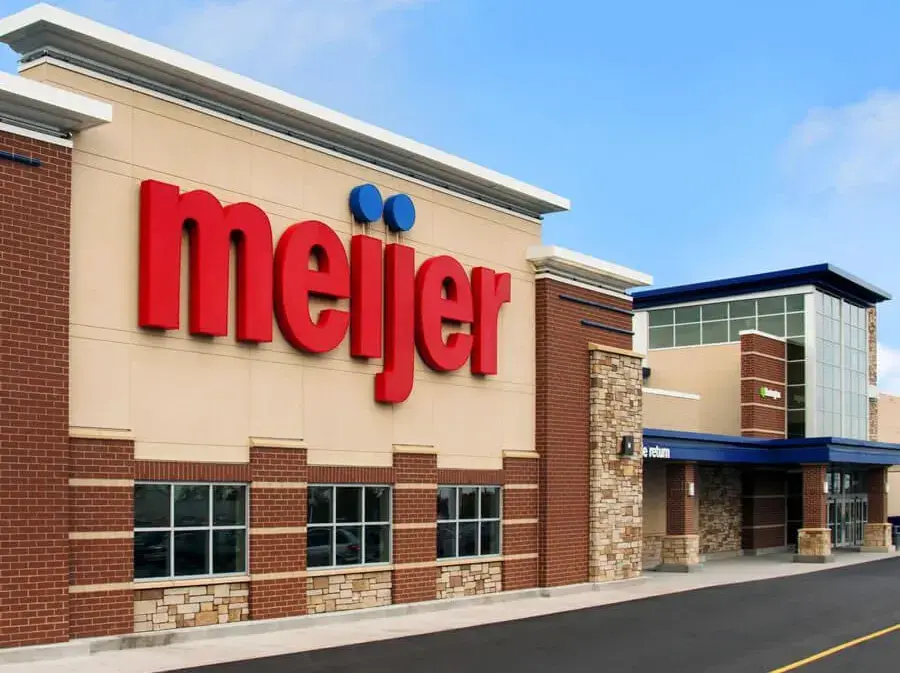
Meijer - Real Estate Predictive Model
Developed a predictive real estate model leveraging XG Boost Regressor, integrating web-scraped market data with existing datasets to forecast daily store visits, achieving a MAPE of 13.3%, enabling strategic retail location decisions.
Project Overview:
As a Data Science Intern (Capstone) at Meijer, I developed a predictive model using the XGBoost Regressor to forecast daily store visits. This model aimed to identify optimal retail locations by integrating web-scraped market data with existing datasets.
Key Milestones:
Data Integration: Combined external data sources like traffic volume, proximity to highways, nearby amenities, and customer sentiment with internal datasets.
Model Development: Leveraged the XGBoost Regressor for predictive analytics, achieving a Mean Absolute Percentage Error (MAPE) of 13.3%.
Impact: Enabled strategic decisions for retail expansion by accurately forecasting store visits, supporting the identification of profitable new locations.
Project Highlights:
Data Collection: Utilized web scraping to gather real-time traffic data and amenities information. Integrated with proprietary data, including demographic insights and competitor analysis.
Predictive Analytics: Focused on key variables such as traffic volume, proximity to highways, and local amenities to enhance the accuracy of location predictions.
Business Implications: Provided a data-driven foundation for strategic retail location decisions, contributing to improved market penetration and profitability.
This project demonstrated the application of advanced data science techniques in retail analytics, offering scalable solutions for optimizing store placement and enhancing customer reach.